The role of AI in predictive climate change modeling
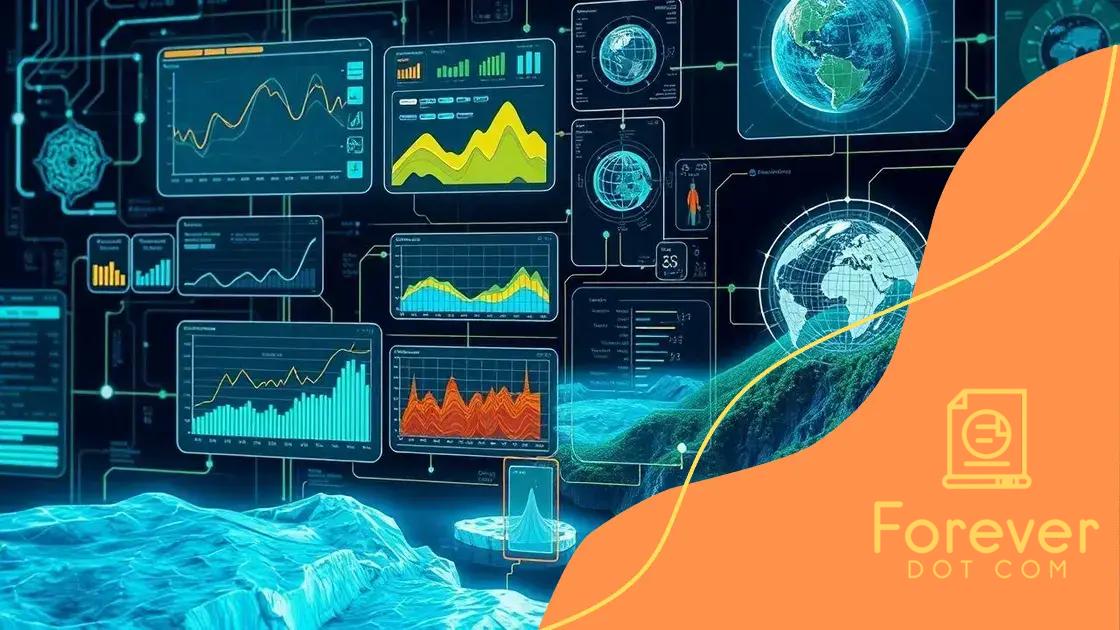
The role of AI in predictive climate change modeling involves advanced data analysis techniques that enhance prediction accuracy, improve disaster management, and support personalized climate solutions to effectively address climate challenges.
The role of AI in predictive climate change modeling is becoming increasingly vital as we navigate environmental changes. Have you ever wondered how technology can help us predict and respond to climate impacts? Let’s dive into this intriguing topic!
Understanding predictive modeling in climate science
Understanding predictive modeling in climate science plays a crucial role in how we assess the future of our planet. This approach allows scientists to simulate various environmental conditions and their potential impacts on the climate. By utilizing data from past and present, they can create models that help us visualize and prepare for changes.
What is Predictive Modeling?
At its core, predictive modeling involves using statistical techniques to forecast unknown outcomes. In climate science, these models can predict temperature changes, sea level rise, and extreme weather events. By analyzing historical data, researchers can make informed guesses about what might happen in the future.
Methods of Predictive Modeling
There are several methods employed in predictive modeling:
- Statistical analysis: Utilizing historical climate data to identify trends.
- Machine learning: Leveraging algorithms to improve predictions over time.
- Simulation techniques: Creating models that mimic real-world conditions.
- Geospatial analysis: Incorporating geographic data to better understand regional impacts.
These techniques work together to provide a comprehensive view of potential climate scenarios. For example, a model might predict how increased greenhouse gas emissions could affect global temperatures over the next century.
Importance of Accuracy
The accuracy of these models is vital. Policymakers and businesses rely on them to make decisions regarding sustainability practices and economic strategies. If the predictions are off, it could lead to significant economic and environmental consequences. Continuous advancements in AI and computing power are helping improve the accuracy of these models, making them more reliable than ever.
As we develop these predictive models, it’s essential to keep updating them with new data. Continuous monitoring and data collection can significantly enhance the accuracy of climate predictions, allowing scientists to adjust their models accordingly and keep up with the rapidly changing climate.
The importance of AI in climate data analysis
The importance of AI in climate data analysis is increasingly recognized as we face more extreme weather events and environmental changes. Through sophisticated algorithms, AI can process vast amounts of climate data, revealing patterns that humans might miss. This capability is essential for making informed decisions related to climate action.
AI Enhances Data Processing
Traditionally, analyzing climate data required significant human effort and was limited by our ability to interpret complex datasets. With AI, we can analyze and interpret these data more quickly and efficiently. For instance, AI can evaluate satellite images and sensor data to monitor deforestation, ice melt, and urban heat islands.
Key Benefits of AI in Climate Analytics
Utilizing AI brings several benefits:
- Increased accuracy: AI models improve predictions by learning from historical data patterns.
- Real-time analysis: Immediate insights allow for faster responses to climate-related events.
- Predictive capabilities: AI helps forecast future climate trends, aiding policymakers in making smart decisions.
- Resource optimization: Enhances the efficiency of energy usage and natural resources.
Moreover, AI can integrate data from multiple sources, including weather stations and ocean buoys, creating a comprehensive overview of climate conditions. This integration is crucial for understanding the interconnectedness of various climate factors.
Challenges and Considerations
While AI offers powerful tools for climate data analysis, challenges remain. For example, not all data is of high quality or accessible. Additionally, interpreting AI outputs requires expertise to ensure that decisions made based on these analyses are valid. Ensuring transparency in AI algorithms is vital, as it builds trust and allows stakeholders to understand the reasoning behind predictions.
In conclusion, as AI continues to evolve, its role in climate data analysis will likely grow, helping humanity better understand and respond to climate challenges. Embracing this technology could make a significant difference in achieving sustainability goals.
Real-world applications of AI in climate forecasting
Real-world applications of AI in climate forecasting are transforming how scientists predict environmental changes. This technology helps analyze patterns, making it easier to foresee weather events and other climate-related issues. As we face increasing risks brought by climate change, leveraging AI becomes essential.
AI in Weather Prediction
One significant application of AI is in weather forecasting. Traditional methods rely heavily on human interpretation of data, which can be limited. AI systems, however, can quickly process vast datasets from satellites and radar. This allows them to generate more accurate weather predictions, helping communities prepare for unexpected weather conditions.
Climate Models Enhancement
AI also plays a vital role in enhancing climate models. By using machine learning algorithms, researchers can improve the accuracy of predictions about long-term climate trends. These improved models can help us understand potential impacts on agriculture, water supply, and biodiversity.
Disaster Response and Management
In the realm of disaster management, AI is crucial. For instance, AI systems can analyze data to predict events such as hurricanes, floods, and wildfires. When integrated with real-time data, AI can assist in determining optimal evacuation routes and strategies, ultimately saving lives.
- Early warning systems: Use AI to monitor environmental changes and alert communities promptly.
- Resource allocation: AI helps in planning and allocating resources efficiently during climate crises.
- Post-disaster analysis: Evaluate the impact of climate events and improve future responses.
Furthermore, companies are using AI to optimize their operations to reduce climate impacts. For example, energy companies can predict demand more accurately, contributing to more sustainable energy management. This blend of AI and practical applications drives innovations essential for addressing climate challenges.
As AI technology evolves, its applications in climate forecasting will continue to grow. This synergy not only supports scientific advancements but also offers practical solutions to mitigate climate change’s effects.
Challenges faced in AI-driven climate models
Challenges faced in AI-driven climate models are critical to understand as we strive for more accurate climate predictions. While AI offers powerful tools, several hurdles remain that can impact the effectiveness of these models.
Data Quality and Availability
One major challenge is the quality and availability of data. AI models rely heavily on large datasets for training and interpretation. If the data is incomplete, outdated, or inaccurate, it can lead to flawed predictions. Gathering high-quality data from various sources, such as satellites, weather stations, and ocean buoys, is essential for building reliable models.
Complexity of Climate Systems
The climate system is inherently complex. It involves numerous variables that interact in unpredictable ways. This complexity makes modeling an accurate representation of real-world conditions challenging.
- Interconnected factors: Elements like ocean currents, atmospheric pressure, and greenhouse gas levels are all interconnected.
- Non-linear relationships: Many climate phenomena exhibit non-linear behavior, complicating predictive accuracy.
- Feedback loops: Some effects can amplify climate changes, creating feedback loops that are difficult to predict.
These complexities mean that even the most advanced AI models may struggle to capture all the nuances of the climate system.
Interpretability of AI Models
Another significant challenge is the interpretability of AI outputs. While AI can produce predictions, understanding how these predictions were derived is often difficult. This lack of transparency can hinder trust among stakeholders such as policymakers and the public.
Furthermore, the need for skilled personnel to interpret AI results adds another layer of complexity. Without adequate training, those interpreting these models may misinterpret or misapply the findings.
Addressing these challenges is essential for improving AI-driven climate models. By focusing on data quality, enhancing interpretability, and recognizing the complexities of climate interactions, we can work towards creating more effective climate prediction tools.
Future trends of AI in climate change adaptation
Future trends of AI in climate change adaptation will play a significant role in how we respond to environmental challenges. As technology continues to evolve, the integration of AI in our strategies for adapting to climate change becomes more critical.
Personalized Climate Solutions
One leading trend is the development of personalized climate solutions. AI can analyze individual behaviors and preferences, providing tailored recommendations for reducing carbon footprints. For instance, smart home systems can optimize energy use based on personal habits, promoting energy efficiency.
Enhanced Predictive Analytics
Another future trend involves enhanced predictive analytics. Improved algorithms will allow for better forecasting of climate-related events. This will enable communities to prepare more effectively for extreme weather, such as floods or hurricanes.
- More accurate models: Advanced AI can improve the precision of models that predict climate impacts.
- Real-time data integration: Using live data feeds for more dynamic adaptation strategies.
- Impact assessments: Allowing for better evaluations of how changes affect ecosystems and human life.
Additionally, AI will be useful in monitoring environmental changes, such as deforestation or land-use practices. Monitoring tools can provide real-time insights, enabling swift interventions and policy adjustments.
Collaboration Across Sectors
Future trends will also emphasize collaboration across various sectors, including governments, NGOs, and private companies. By sharing data and insights, stakeholders can develop comprehensive approaches to climate adaptation. AI can facilitate these partnerships by analyzing shared data and identifying common needs.
As AI technology advances, its capacity to assist in climate change adaptation will likely expand. Innovations in machine learning and data analytics will create new tools that make it easier to adjust and respond to the changing environment.
FAQ – Frequently Asked Questions about the Role of AI in Climate Change Modeling
How can AI improve climate predictions?
AI can analyze vast amounts of climate data quickly, revealing patterns that enhance the accuracy of weather forecasts and climate models.
What challenges does AI face in climate science?
AI faces challenges such as data quality, the complexity of climate systems, and the need for transparency in model outputs.
How is AI used in disaster management?
AI helps predict extreme weather events and optimize response strategies, allowing for quicker and more effective disaster management.
What future trends can we expect from AI in climate adaptation?
Future trends include personalized climate solutions, enhanced predictive analytics, and greater collaboration between sectors to address climate challenges.