How machine learning is improving supply chain efficiency
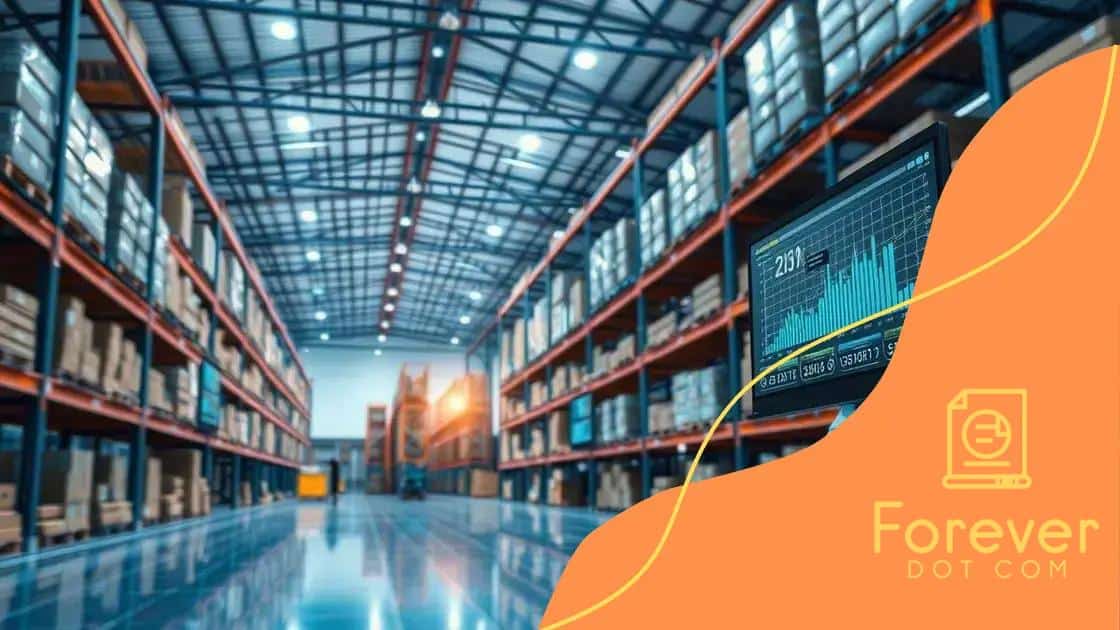
Machine learning is improving supply chain efficiency by optimizing demand forecasting, enhancing operational processes through automation, and enabling real-time data analysis for better decision-making.
How machine learning is improving supply chain efficiency is a topic worth exploring as it reshapes logistics operations globally. Have you ever wondered how data analysis can speed up deliveries and cut costs? Let’s dive into the transformative impact of machine learning.
Understanding machine learning in supply chains
To grasp how machine learning integrates into supply chains, it’s essential to recognize its core principles. Essentially, machine learning encompasses algorithms that allow computers to learn from data, improving their accuracy in predictions and processing. In the context of supply chains, this translates to large volumes of data being examined to identify patterns.
Understanding machine learning in supply chains can lead to significant improvements in efficiency and productivity. As companies collect data from various sources, machine learning helps streamline operations by analyzing trends and optimizing processes.
Key Components of Machine Learning
Machine learning consists of several components that work together to enhance supply chain efficiency:
- Data Collection: Gathering accurate data from multiple sources is crucial.
- Data Processing: Transforming raw data into usable formats makes it easier to analyze.
- Algorithm Selection: Choosing the right algorithm is vital for making accurate predictions.
- Model Training: Training models on historical data helps improve future forecasting.
By utilizing these components, businesses can make informed decisions based on real-time data. For instance, imagine a logistics company leveraging machine learning to predict delivery times more accurately. This not only helps in scheduling deliveries but can also enhance customer satisfaction as clients receive their products on time.
Real-World Applications
Many industries are currently harnessing machine learning to revolutionize their supply chains. Retailers, for example, utilize predictive analytics to optimize their inventory levels. This ensures they have the right amount of stock available at the right times, minimizing waste and maximizing sales.
Furthermore, manufacturers are employing machine learning for proactive maintenance of equipment. By analyzing data from machines, they can predict failures before they occur, thus avoiding costly downtime. This kind of precision leads to smoother operations and is becoming increasingly important in today’s competitive market.
The beauty of machine learning lies in its adaptability. As more data is collected, the systems improve over time. This establishes a continuous cycle of enhancement, ensuring that companies remain agile and responsive to market changes.
Key benefits of machine learning for logistics
Exploring the key benefits of machine learning for logistics shows how this technology changes operations for the better. With machine learning, logistics companies can use data to make smarter decisions and streamline their processes.
One major benefit is improved demand forecasting. Machine learning algorithms can analyze past data to predict future trends better than traditional methods. This leads to more accurate inventory management. As a result, businesses can reduce excess stock and avoid shortages.
Enhanced Operational Efficiency
Machine learning also plays a vital role in enhancing operational efficiency. By optimizing routing for delivery trucks, logistics companies can minimize fuel consumption and reduce delivery times.
- Cost savings: Efficient routing means less fuel and lower operational costs.
- Time management: Reducing delivery times boosts customer satisfaction.
- Data-driven decisions: Machine learning offers insights that facilitate quick adjustments in operations.
Another advantage is the improvement in warehouse management. Machine learning can assist in managing stock levels and predicting when to reorder. This minimizes human error and frees up team members for more strategic tasks.
Moreover, supply chain transparency increases through machine learning. Companies can track shipments in real time, making it easier to respond to delays or issues as they arise. This level of oversight enhances communication between suppliers, carriers, and customers.
Overall, machine learning creates a smarter logistics environment. Companies that leverage these technologies can stay competitive and effectively meet customer demands while saving time and resources.
Real-world examples of machine learning applications
Exploring real-world examples of machine learning applications helps us understand its impact across various industries. Companies are increasingly turning to machine learning to solve complex problems and improve their services.
In the retail sector, for instance, major brands use machine learning for personalized recommendations. By analyzing purchase history and user behavior, these brands can suggest products that customers are more likely to buy. This not only enhances user experience but also drives sales growth.
Examples in Different Industries
Machine learning is transforming logistics, finance, healthcare, and many more fields. Here are a few notable applications:
- Logistics: Companies like Amazon use machine learning for optimizing supply chain routes. This reduces delivery times and improves customer satisfaction.
- Finance: Banks utilize machine learning to detect fraudulent transactions. Algorithms can analyze transactional data in real time, flagging any suspicious behavior.
- Healthcare: Hospitals apply machine learning to predict patient outcomes. By analyzing patient data, they can find patterns that help them provide better care.
- Manufacturing: Factories use machine learning for predictive maintenance. Machines are monitored to predict failures before they occur, minimizing downtime.
Furthermore, the transportation industry benefits from self-driving technology. Companies like Tesla use machine learning algorithms to enable vehicles to learn from their environments and make driving decisions. This innovation promises to revolutionize how we think about transportation.
Each of these examples demonstrates how machine learning is not just a buzzword but a practical tool. It streamlines processes and offers solutions that were once thought to be futuristic.
Challenges faced in implementing machine learning
While the advantages of machine learning are clear, there are several challenges faced in implementing it effectively. Many organizations encounter obstacles that can slow down their adoption of this technology. Understanding these challenges is crucial for successful integration.
One significant issue is the quality of data. Machine learning algorithms rely on accurate data to make predictions. If the data collected is flawed or incomplete, it can lead to incorrect outcomes. Ensuring data integrity and quality is vital to derive meaningful insights.
Technical and Skills Gap
Another challenge pertains to the technical skills required. Many businesses struggle to find professionals with the necessary expertise in machine learning. This skill gap can hinder the ability to implement and maintain machine learning systems effectively.
- Recruitment difficulties: Finding qualified data scientists can be challenging.
- Training existing staff: Upskilling employees may require significant time and resources.
- Rapid technological changes: Keeping up with evolving technologies can be overwhelming.
Additionally, organizations may face high costs associated with integrating machine learning solutions. From software and hardware investments to training personnel, the overall expenses can be substantial. These financial barriers can be a significant deterrent for small and medium-sized businesses.
Cultural resistance is another challenge that can arise. Employees may be hesitant to adopt new technologies due to fear of change. This reluctance can create friction within teams and stall progress if not addressed properly.
As companies navigate these obstacles, it is essential to develop a clear strategy and foster a culture that embraces innovation. By understanding the challenges, organizations can proactively work to mitigate them, paving the way for successful machine learning implementation.
Future trends in supply chain technology
Examining future trends in supply chain technology reveals exciting developments that could shape industries. As technology continues to advance, logistics and supply chain management are set to evolve significantly.
One major trend is the increased integration of artificial intelligence and machine learning. Companies are looking to leverage these technologies to enhance efficiency and improve decision-making. AI can analyze vast amounts of data, enabling more accurate predictions and better responses to market fluctuations.
Automation in Supply Chains
Another notable trend is the rise of automation. Automation technology, including robotics and drones, will play a crucial role in streamlining operations. Businesses can use automated systems for tasks like inventory management and order fulfillment.
- Robotic process automation: Reduces manual errors and speeds up processes.
- Drones: Can expedite deliveries, especially in hard-to-reach areas.
- Warehouse automation: Enhances efficiency in stock handling and decreases labor costs.
Additionally, the Internet of Things (IoT) is becoming increasingly vital in supply chains. IoT devices can monitor equipment and track shipments in real time, providing valuable insights into the entire supply chain process. This connectivity allows companies to respond swiftly to issues and optimize their operations.
Furthermore, blockchain technology is gaining traction within supply chains. It offers enhanced transparency and security in transactions. By using blockchain, businesses can track products from origin to delivery, ensuring authenticity and reducing fraud. This trend is particularly relevant for industries where provenance matters.
As we look to the future, sustainability will also shape supply chain strategies. Companies are focusing on green technologies and practices to reduce their carbon footprint. This shift towards sustainability is not just a trend but a necessity for staying competitive in a market that increasingly values environmental responsibility.
FAQ – Frequently Asked Questions about Machine Learning in Supply Chains
What are the main benefits of using machine learning in supply chains?
Machine learning improves demand forecasting, enhances operational efficiency, and allows for real-time tracking of shipments.
How can companies ensure data quality for machine learning applications?
Companies can ensure data quality by implementing rigorous data management practices and using accurate data collection tools.
What challenges do businesses face when implementing machine learning?
Common challenges include data quality issues, skill gaps among employees, high initial costs, and resistance to change from staff.
How do future trends like blockchain impact supply chains?
Blockchain technology enhances transparency and security in transactions, allowing businesses to track products accurately from origin to delivery.